Disclaimer: This is part of my notes on AI research papers. I do this to learn and communicate what I understand. Feel free to comment if you have any suggestion, that would be very much appreciated.
The following post is a comment on the paper The Era of 1-Bit LLMs: All Large Language Models Are in 1.58 Bits by Shuming Ma, Hongyu Wang, Lingxiao Ma, Lei Wang, Wenhui Wang, Shaohan Huang, Li Dong, Ruiping Wang, Jilong Xue, and Furu Wei.
Ma et. al. from Microsoft Research Asia and University of Chinese Academy of Sciences, are developing a new research line on 1-bit Large Language Models (LLMs) under the frame of General AI, whose mission is to advance AI for humanity. This is the second paper they have published in this regard.
This work is a follow up on ther previous publication where they introduced BitNet. The project is motivated by the increasing size of LLMs that pose challenges for deployment and raise concerns about the environmental impact due to the high energy coonsumption.
Authors claim that the new version introduced in this paper, BitNet b1.58 provide a Pareto solution to reduce inference cost of LLMs while maintaining the model performance. Thus, BitNet b1.58 enbales a new paradigm of LLM complexity and calls for actions to design new hardware optimized for 1-bit LLMs.
BitNet b1.58
The main difference is in regard of the binarization step. There is no binarization anymore, now they adopt absmean quantization where the weights are set to be $+1, -1$ or $0$. This step is formalized as:
\begin{align} \tilde{W} &= \text{RoundClip}(\frac{W}{\gamma + \epsilon}, -1, +1), \\ \text{RoundClip}(x,a,b) &= \max(a,\min(b,\text{round}(x))), \\ \gamma &= \frac{1}{nm}\sum_{i,j}|W_{i,j}|. \end{align}
The rest of the BitLinear layer remains the same as in the original BitNet.
A good thing that they mention is that the method incorporate LLaMA-alike components so it is easier to implement them into the open-source frameworks.
Results
There is a dramatic improvement in terms of energy consumption and memory usage, while maintaining state-of-the-art performance. The following figures ilustrate this.
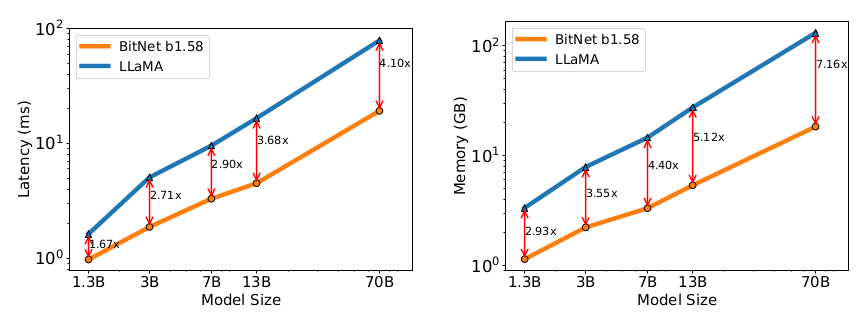

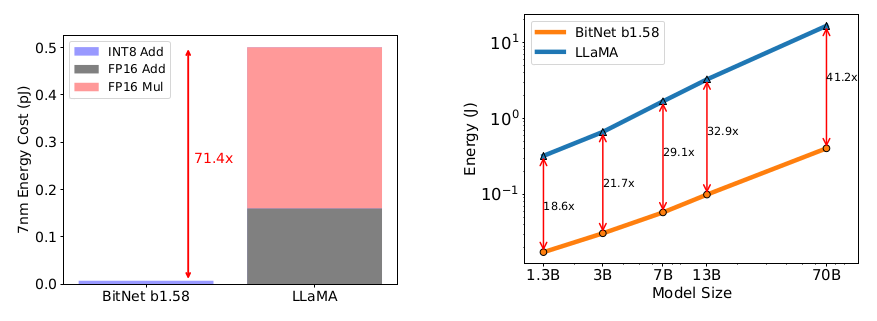

Personal thoughts
I believe this paper is a game changer. They have unveiled a new paradigm, this is not only a new era of LLMs, but a new era of DNNs. I want to see 1-bit everything and everywhere! 1b-ResNet, 1b-YoLo, 1b-CNNs, etc.
Whenever I see binary something I automatically think about sparsity. Is it possible to make the active weights sparse so that the model can benefit from sparsity to make it even more efficient?
Another thing that I already commented in the previous post about BitNet, is whether it would be possible to also binarize the data. Encoding the inputs into a binary space then performing binary operations with binary weights and finally debinarizing to get the sampling probabilities.
References
[1] Ma, S., Wang, H., Ma, L., Wang, L., Wang, W., Huang, S., Dong, L., Wang, R., Xue, J., & Wei, F. (2024). The Era of 1-Bit LLMs: All Large Language Models Are in 1.58 Bits. arXiv:2402.17764.
[2] Wang, H., Ma, S., Dong, L., Huang, S., Wang, H., Ma, L., Yang, F., Wang, R., Wu, Y., & Wei, F. (2023). Bitnet: Scaling 1 Bit Transformers for Large Language Models. arXiv:2310.11453.